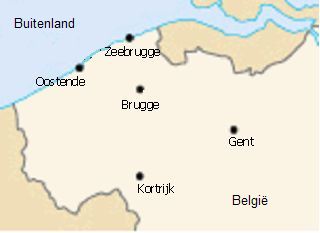
Pages
UGent - Cancer Research Institute Ghent (CRIG)
Abstract advanced bachelor of bioinformatics 1 2019-2020: Identification of candidate drug targets for the treatment of pediatric tumors
Neuroblastoma is an aggressive embryonal tumor of the sympathetic nervous system in children, which can regress spontaneously or grow and metastasize with resistance to multiple therapies. Neuroblastoma is characterized by DNA Copy Number Alterations (CNAs). Patients with neuroblastoma are categorized in different risk groups depending on the characteristics of the tumor. There are several risk groups(High and Other risk) and only a part of these risk groups only does show MYCN amplifications.
There are already some oncogenic driver genes identified (such as MYCN). Yet many driver genes remain to be found on the DNA CNA’s. In order to detect these driver genes, RNA expression and clinical data using multi-omics network inference are used. These potential novel drivers and their regulators can open a new approach for targeted therapy.
The starting point for this investigation is the clinical and RNA-expression data of 497 patients. In my traineeship my task was to analyze this dataset in pre-and postprocessing for network inference. This dataset had several clinical variables (including Risk group and MYCN amplification). Using the R(studio) software (edgeR package) a differential expression analysis (DE-analysis) was performed. This included several pre-processing steps including (log)-transformation, filtering, normalization and clustering of the dataset. The filtering was performed by only selecting the genes that were expressed in at least three samples. The normalization was performed using the Trimmed Mean of M-values method. For the clustering step both MultiDimensional Scaling and Principle Component Analysis were performed. After the DE-analysis between the high risk MYCN amplified and high risk non-MYCN amplified patients, no differentially expressed genes could be found.
The next step was to perform a feature extraction of genes. for network inference. By extracting highly variable genes, we retain as much information for statistical learning, while removing possible noise. To be able to perform this a certain variance cut-off value was to be determined. Using a histogram plot of the variances, we chose a cut-off value of 0,5. I also prepared “regulator lists” for the network inference, using several databases such as humanTFDB ( as a part of the animalTFDB), the CR2Cancer, Epifactors database and the Ensembl database. This involved data downloading, data wrangling for correct gene identifiers and only retaining the non-redundant genes with expression info in the data.
For post-processing, I focused on functional annotation using Gene Set Enrichment Analysis (GSEA) of the genes, after the DE-analysis and after the network inference on a set of co-expression/coregulatory modules. GSEA often starts with a preranked list of genes based on logfold changes. The Cluster profiler package in R and the MSigDB were used to perform the GSEA. This is the latest step in the investigation and these results are in the process of being interpreted.
Tags: bioinformatics |
Address
Sint-Pietersnieuwstraat 25
9000 Gent
Belgium |
Contacts
Traineeship supervisor
Vanessa Vermeirssen
09/26423945 vanessa.vermeirssen@ugent.be |