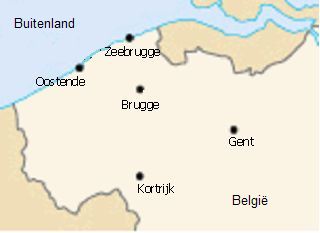
Pages
OncoRNALab - Centre for medical genetics Ghent
Abstract 2020-2021: COMPARISON OF CRISPR SGRNA OFF-TARGET PREDICTION ALGORITHMS
The use of CRISPR interference (CRISPRi) technology makes it possible to knockdown target gene expression without altering the DNA sequence and relies on two main components: a guide RNA (gRNA) and a deficient CRISPR associated nuclease 9 (dCas9). The gRNA is a specific complementary RNA sequence that recognizes the target DNA region of interest and directs the dCas9 nuclease there to block transcription (on-target effect). However, sometimes the single guide RNA (sgRNA) can lead to off-target effects because mismatches between the sgRNA and target site can lead to aspecific binding in the genome, depending on the number of mismatches and their position(s). This means that the sgRNA will target another place in the genome, and thus the wrong place.
The goal of this project is to evaluate off-target prediction tools in how “good” they can predict these off-target effects and to accomplish this goal the project is divided in three major parts. The OncoRNALab has generated RNA-sequencing data for more than 200 sgRNA’s covering 24 targets which are individually delivered to HEK293T-cells.
In the first part, the High Throughput Sequencing (HTS) count tables, generated out of the RNA-sequence data of the sgRNA knockdown experiments, are used to perform a differential expression analysis (DEA) between the sgRNA conditions and the negative controls. This is done with the DESeq2 package, of Bioconductor, in R. The HTS count tables of all the sgRNA samples are used to create a “DESeqDataSet”. This dataset contains 58.471 genes and 276 sgRNA samples. A pre-filtering on this dataset makes sure that only genes with a minimum of 5 counts in at least 50 % of the samples are kept. After this, expression data of 13.535 genes remain and is used for the DEA. After the DEA, a list of differentially expressed genes (DEG) for each one of the samples is generated.
Second, an off-target prediction tool (Cas-OFFinder) is used that based on the sgRNA sequences searches for potential off-target sites of Cas9 RNA guided endonucleases, given a certain number of mismatches. For this project, off-target gene lists were created with 0, 1, 2 or 3 mismatches.
In the last part of the research project, the comparison between the observed transcriptome changes (DEG list) and the expression changes of the predicted off-target gene list is performed in R. For this step, it was necessary to determine the closest up or downstream gene and a window of 50kb and 500kb with the predicted off-target positions, provided by the tool. The comparison is done by creating a density plot that shows the log2 fold change of all the genes, from the DEG list in the first part of the project, in overlay with a density plot of the log2 fold change of the off-target predicted genes. A shift in density on the log2 fold change towards downregulated genes will indicate if there is an overrepresentation of off-target effects.
Density plots were made for a 50kb window and a 500kb window. Four plots were made for both windows, depending on the number of mismatch numbers (0, 1, 2 or 3). So, in the end eight density plots were constructed. The results of the different density plots showed no shift in density of the log2 fold change. In fact, all density plots were identical. This may be because the off-target genes are not expressed and are therefore filtered out of the dataset due to too low counts, or it may be that the genes are just not picked up during RNA-sequencing. If this would be the case, it may mean that the design of the sgRNAs in the CRINCL library are of good quality. This pipeline can therefore also be used (with minor adjustments) to analyze other off-target prediction tools and then compare them with the current results of the Cas-OFFinder.
Tags: bioinformatics |
Address
Belgium |
Contacts
Traineeship supervisor
Eric De Bony De Lavergne
Eric.deBonydeLavergne@UGent.be |
Traineeship supervisor
Wim Trypsteen
wim.trypsteen@ugent.be |
|