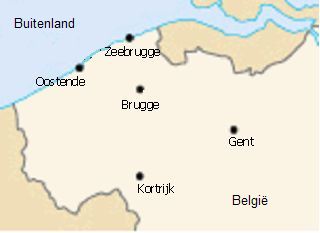
Pages
UG, vakgroep (micro)biologie
Stageonderwerp 2010-2011:
Stageonderwerp 2009-2010: diversiteit van bacteriële symbionten in het groenwier Boergesenia
|
Stageonderwerp 2005-2006
c) Amplificatie van het volledige cp genoom door rolling circle amplification (RCA): isotherme amplificatie dmv bacteriophage Ph29 polymerase, in staat tot DNA synthese van meer dan 70 kb. Deze techniek werd vooral gebruikt voor amplificatie van het menselijke genoom en voor dit doel is er een commerciële kit voorhanden.
To study the taxonomy and epidemiology of bacterial pathogens it is important to obtain as much information as possible about these strains. As sequencing technology has become more advanced and cheaper it has become custom to sequence whole-genomes instead of only a few individual genes. However, for many bacterial species there is no reference genome available yet. The aim of this project was to process raw whole-genome sequencing data to create assembled and annotated genomes that can be published and used as a reference for future research.
Two different datasets were used, i.e. one with 52 Achromobacter species and one with 19 Burkholderia species. Both datasets consist of raw data in fastq.gz format that was generated by the Illumina HiSeq 4000 and Illumina NovaSeq 6000 platforms. A pipeline was followed to turn these reads into assembled and annotated genomes. The first step was to evaluate the quality of the raw data and to filter them on a minimum read length of 50bp and a quality score of Q30. This was done with fastQC and fastp. Next, we used Shovill which is a tool that combines SPAdes, bwa, samtools and pilon. The amount of reads are by default downsized to a subset with a sequencing depth of 150. SPAdes assembled the genome by using Kmers according to the De Bruijn graph principle, which resulted into the construction of contigs. They were then filtered on a minimum contig length of 500bp. The raw reads were mapped against these contigs with bwa and sorted and indexed with samtools. Pilon then performed an alignment analysis and tried to make improvements to the assembly.
The assembled genomes were annotated with Prokka. To check the quality of our final assemblies, we mapped the raw reads against their assemblies and used Qualimap to provide us more details about the features of the resulting alignment. Important checks are the amount of unmapped reads and coverage. CheckM is another method that was used to evaluate the quality of the annotated assemblies by estimating if the genome is complete or possibly contaminated. As a second part in the project, housekeeping genes were extracted from the genomes and added to an internal database. Multi-Locus Sequence Typing analysis was performed to determine the sequence type of the strains. Because there is variation in the sequence of these housekeeping genes between different strains, this kind of information is important for epidemiological studies.
For both datasets one housekeeping gene was used as a control to verify the authenticity of the samples. The sequence that was extracted from our genome was compared via multiple sequence alignment to the sequence of a gene that was previously determined from the DNA sample via Sanger sequencing. For this MUSCLE was used in MEGA7 software. A phylogenetic tree was also constructed using the Neighbour-Joining method to visualize the evolutionary relationships. In figure 1 we can see that the sequences obtained via Sanger sequencing and via whole-genome sequencing (indicated by “exseq WGS”) cluster together for each of the samples.
Finally bcgTree was used to make an extended phylogenetic tree based on maximumlikelihood analysis of 107 different genes from the strains of both datasets. The genomes that passed all quality controls were approved for publication. Both the raw reads and the annotated genomes were registered and uploaded into the European Nucleotide Archive. As a result, 41 Achromobacter and 15 Burkholderia strains are now publicly available through the accession numbers PRJEB37567 and PRJEB37806, respectively.
Background: The project I’m working on is BioSoCr, a project of the research group PAE, part of Biology Section. It is a project that investigates the “Greening of the Arctic”, which is probably a direct correlation with the warming of the climate. For this there were samples taken in Svalbard, an island very close to the north pole. Samples were taken at 12 different location. At those locations there were samples taken at three positions: Young (no vegetation), Intermediate (small vegetation) and Old (lot of vegetation), and there were samples taken at the top layer, and deeper (5-10cm). Also tree biological replicates were taken per place, called A-B-C. The aim of my traineeship is to perform the bio-informatics analysis on the first set of samples.
Method: First I started with the raw analysis of the samples. I checked for quality, ran a “multiqc” to check for the quality. After that I assembled the sequences, using the PEAR program, then performed a quality filtering using USEARCH. After that I merged the samples and only kept the unique sequences, on which I checked for chimeras using USEARCH. At last the blast analysis was performed using the program MOTHUR. After that there was a manual check of the data, to check for contamination, delete the organisms that are not interesting for the project, …
Then I performed the further analysis in R. Within R I first started with the analysis of the raw data, check the library sizes, look at the rarefaction curve and check for the amount of reads I loose after filtering. Based on the analysis of the library sizes, the decision is made to normalize the Eukaryotic libraries to 3500 reads and the Prokaryotic libraries to 10000 reads. The reason for this difference is because I lost a lot of reads for the Eukaryotes after deleting the Embryophyceae. For the other parts of the R analysis we worked with the normalized data.
Second part was performing some statistical analysis on the data, performing an NMDS analysis, Heatmap and CAP analysis. In the CAP analysis you include some metadata in the calculation. For our dataset, I checked for clustering by both including the metadata “Location” and metadata “Deep/Top” in the CAP analysis.
In the last part I looked more into detail at the different taxonomic groups which were available in the samples. I checked the overall biggest groups for the Eukaryotes and Prokaryotes, then checked per location what were the differences. Also a dendogram was made to check for the most diverse groups of organisms. At last the most interesting groups were checked for their composition in more detail.
Results: For the statistical analysis, NMDS was least informative, CAP and Heatmap were giving better results. For the NMDS I could not find a real clustering of the samples, based on some metadata. For the CAP analysis with both location and depth of sampling there was a nice clustering visual. This was also statistically substantiated with the CCR (correct class ratio) values. Also Heatmap was showing that the samples had some similarity. The similarity was biggest in the 16S samples, where the Heatmap was calculate based on the presence/absence of the different OTU’s.
For the taxonomic analysis I could find the major groups of Prokaryotic and Eukaryotic organisms and found that the biggest groups of Prokaryotes were Proteobacteria, Actinobacteria and Acidobacteria. For the Eukaryotes I found that the biggest groups were Opistokonta, Achaeplastida and Alveolata.
Conclusion: As a main conclusion of the different parts I could state that for the first part of the analysis, the analysis of the raw data, I had to make some changes in the script, but overall it was very well documented. For the future though, I would want to include some other pipeline, to be able to compare.
As for the work in R, I first looked at the correlation of the samples and if the different metadata were clustering together yes or no. For that I tried several options, an MDS, NMDS, PCA, CAP analysis. At the end I only kept NMDS and CAP analysis. The advantage of NMDS compared with MDS is that NMDS in more robust. PCA was left out, because this method is not so compatible with a lot of zero-counts. CAP analysis has come out as the best one, because this analysis can include the different metadata you want to correlate. It is also statistically substantiated. It gives the CCR (correct class ratio) which indicates the “correctness” of the clustering. At last I also included a heatmap, this gives the correlation between the samples with a color code, which also gives a good representation of how related samples are. The last part in R included some taxonomic analysis. This was very important to look further into detail at the different taxonomic groups, which ones appear the most, are there a lot of differences in between location, in between sampling depth, … Of course for this last part, there are further analysis necessary to loopback to the original set-up of this project. Is what we find logical, compared to what is expected.
Tags: biology; bioinformatics |
Address
KL Ledeganckstraat 35
9000 Gent
Belgium |
Contacts
Traineeship supervisor
Olivier De Clerck
09 264 8500 olivier.declerck@ugent.be |
Traineeship supervisor
Frederik Leliaert
09 264 8508 frederik.leliaert@ugent.be |
Traineeship supervisor
Heroen Verbruggen
09 264 8507 heroen.verbruggen@ugent.be |
Traineeship supervisor
Bjorn Tytgat
092648504 bjorn.tytgat@ugent.be |
Traineeship supervisor
Charlotte Peeters
0924645128 charlotte.peeters@ugent.be |
|