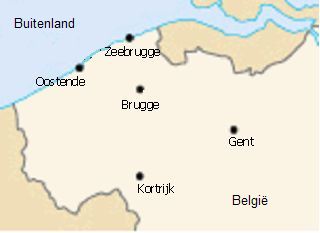
Pages
Kulak, Public Health and Primary Care
Abstract traineeship advanced bachelor of bioinformatics 2019-2020: A comparison of methods for Transposable Elements Classification
Transposable elements (TEs) are DNA sequences that are capable of moving and creating copies of themselves within the eukaryotic genome. They cause genetic variability and promote changes in the genes’ functionality. Transposable elements are the most represented sequences within the eukaryotic genome. These transposable elements are classified in a hierarchical way into classes, subclasses, orders and superfamilies according to Wicker’s taxonomy. The classification of these TEs is important to understand their role in the genome since each order has a different function and a different way of copying themselves.
During my internship at KULAK, I searched for methods to classify these TEs. This is done using two different methods: Homology methods and machine learning methods.
Homology methods have been around for a long time and are well researched. These methods use prior information such as TE libraries. These TE libraries are often included in the program or tool that performs the homology method. It is important that these TE libraries are classified according to Wicker’s taxonomy. Another important factor in these homology methods is that a training set can be used. This usually improves performance.
Machine learning methods create algorithms that learn automatically. There are different types of machine learning that can be used to find TEs, such as neural networks and hierarchical classification. Machine learning methods have proven to be promising in the search for transposable elements, because they can are able to use the different properties of TEs such as length, ORFs and motifs to classify instances into the correct superfamily.
Despite the fact that both homology methods and machine learning methods are well researched, there is very little comparison between these two field.
The goal of my internship is to search for homology methods and machine learning methods that can be used to classify and identify TEs. These methods would be performed on public repositories that contain TEs. After performing both methods, the results would then be compared. The most important criteria for these results are the number of correctly classified instances and the speed at which the method works.
Abstract traineeship advanced bachelor of bioinformatics 2017-2018: Benchmark datasets for interaction datamining
The CLUS tool developed at KU Leuven contains a Hierarchical multi-label classification (HMC) algorithm capable of predicting multiple, hierarchically organized classes for a protein at the same time. The algorithm was evaluated using different train, test and validation datasets. These datasets consist out of multiple experimentally derived attributes for all yeast proteins, and their classifications in 2007 derived from either MIPS Functional Catalogue (FunCat) (http://mips.helmholtz-muenchen.de/funcatDB/) or Gene Ontology (GO) (http://www.geneontology.org/ ). To check the predictive capabilities of the program we have updated the datasets with the current classifications according to the FunCat and Gene Ontology databases. To do this, current classifications were retrieved from GO and FunCat in an automated way using R. As such the old classifications were replaced with the new ones, while keeping old classifications for comparison purposes. Comparing the predictions CLUS makes for a given protein based on the old classifications, with the newly annotated classifications in MIPS and Gene Ontology illustrates the predictive ability of the algorithm. The updated train, test and validation sets can also be used to retrain the algorithm for more accurate predictions based on the updated knowledge in MIPS and GO.
The second part of this internship concerns the STITCH database. This involves the interactions of proteins and chemicals. We have created an interaction file containing the interaction between a chemical and every protein in the database with the score according to STITCH. Another file holding fingerprint information for the chemicals was generated using the OpenBabel software (http://openbabel.org/wiki/Main_Page ). These fingerprints are a numeric representation of different informative features of a chemical, allowing for easy comparison between them. To allow comparison between the proteins, both Pfam(https://pfam.xfam.org/ ) and GO ID’s were retrieved and converted to a binary vector. By comparing fingerprint vectors between chemicals and binary Pfam/GO vectors it’s possible to predict interactions of interest between proteins and chemicals which can then be experimentally verified.
Tags: bioinformatics |
Address
Etienne Sabbelaan 53
8500 Kortrijk
Belgium |
Contacts
Traineeship supervisor
Celine Vens
celine.vens@kuleuven.be |
Traineeship supervisor
Felipe Kenji Nakano
493 14 34 25 felipekenji.nakano@kuleuven.be |
|