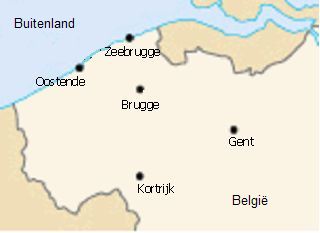
Pages
UZ Gent centrum voor medische genetica
Abstract Bachelor Project FBT 2021-2022: Extending gene panels to detect mutations in tumor suppressor genes via hybridization- based NGS
Next generation sequencing (NGS) is the most used technique in molecular diagnostics to detect variants in DNA. In the Centrum Medical Genetics Ghent (CMGG) they have several gene panels to detect pathogenic variants or variants associated with an increased risk to develop certain disorders. The aim of this study is to extend the existing gene panels with genes that are now examined in a more labor-intensive way. The probes that are used to sequence the panel are XGen® Lockdown® probes from Integraded DNA Technologies.
Before the genes can be added to the routinely used the panels, they be validated. This starts with a DNA extraction performed by a MagCore® Automated Nucleic Acid Extractor, then the concentration of the DNA is measured. After that, a library preparation (HyperCap Roche) is performed. The library prep starts with an enzymatic fragmentation followed by adaptor ligation. Then the library and probes are mixed together, to hybridize. Lastly magnetic streptavidin coated beads are used to capture and clean-up the hybridized probes. The selected fragments are diluted to a 4nm pool that can be sequenced. Therefore the MiSeq or NovaSeq 6000 is used.
The first NovaSeq 600 generated a large number of reads for each sample. Therefore every region had high coverage. As such high coverage is not feasible in routine diagnostics, the pools were diluted 1/10 to decrease the number of reads per sample. With the lower number of input reads some regions did not have enough coverage. We found several reasons why some of the exons have insufficient coverage. Most of them are not completely covered by the probes, GC- rich and have short repeats. The on-target rate of the total panel is expected to be higher than 30%. In most of the cases this was not achieved. A possible explanation for this are the repeats, which can be mapped wrong. Also the detected variants were compared with the variants that are found in the routinely used test. There were two variants that couldn’t be detected and this should be further explored. Also possible false-positive variants were found, but further research is necessary to confirm this. An optimisation of the used probes is also recommended to increase the coverage.
Abstract Bachelor Project 1 FBT 2020-2021: IMPLEMENTATION OF DIGITAL MULTIPLEX LIGATION-DEPENDENT PROBE AMPLIFICATION IN A DIAGNOSTIC SETTING
This bachelor project handles on the implementation of digital Multiplex Ligation-dependent Probe Amplification (dMLPA) in a diagnostic setting. Manual Multiplex Ligation-dependent Probe Amplification (MLPA) is currently used for diagnostic purposes, but this rather labor intensive. Therefore Center for Medical Genetics Ghent wants to implement dMLPA in a diagnostic setting. With MLPA and dMLPA (multi) exon deletions and duplications can be detected. Deletions and duplications can be involved in the hereditary predisposition of different types of cancers. dMLPA makes it possible to analyse multiple genes in one experiment with the help of barcodes, whereas MLPA can only look at one or two gene(s) in parallel.
Before dMLPA can be implemented, test runs are need to be done with this technique and the data need to be carefully analyzed. The deletions and duplications that are detected in the data are first confirmed with a manual MLPA. If the manual MLPA can confirm the deletions or duplications, the breakpoints are further investigated. Therefor primerdesign is carried out that overlap the region where the deletion or duplication occurs. A polymerase chain reaction (PCR) is used to evaluate whether the primers induce amplification of the patient sample. To check the amplification the Fragment Analyzer is used, eventually the PCR product is sequenced using Sanger sequencing. Finally by using FinchTV or SeqPilot the breakpoints can be located and may confirm the results of the dMLPA.
Seven runs have been run with the dMLPA, with a total of 250 samples. The obtained results are further evaluated. 34 (13.6 %) (multi) exon deletions and 14 (5.6 %) were duplications. 8 % of the samples dropped out, due to various reasons. The reasons could include low quality of the DNA sample the failure of probe hybridization. All deletions and duplications except one were subsequently confirmed with a manual MLPA. The sample not confirmed with the manual MLPA can be considered as error called during the result analysis of the dMLPA. In 17.6% or six deletions, a variant was found under the probe, 8.8% or three deletions were considered false positive as no variant or deletion was found and 5,9 % or two breakpoints of a multi-exon deletion were found. Duplications were not further investigated in detail in this bachelor project, this will be investigated in the near future.
Abstract Bachelor Project 2 FBT 2020-2021: Investigating the role of zebrafish as animal model for Loeys-Dietz syndrome type III and bicuspid aortic valve
Background: The genes SMAD3 and SMAD6 have been linked with hereditary human diseases, such as HTAD, bicuspid aortic valves, and Loeys-Dietz syndrome type III. Based on clinical evidence, this study plans to evaluate the role of SMAD3 for vascular diseases, such as pathologic dilation of the aorta (aneurysms), ectopic blood flow between the layers of the aorta, and craniosynostosis caused by SMAD6 (protein) deficiency.
Aim: The main goal of this study is to look at the cardiovascular and skeletal phenotypes of zebrafish models with different combinations of SMAD3 and SMAD6 knockout mutations in order to better understand their role in disease development.
Methods: Molecular biology techniques such as Sanger sequencing and alizarin red staining were used to genotype and phenotype for this bachelor project. Ultrasound imaging is used to research cardiovascular development in adult zebrafish. The Kaplan Meier Curve is used to investigate the correlation between genotypes and survival rates.
Results: The survival curve revealed a sudden collapse around 12 days post fertilization for most genotypes observed. A significant increase in the number of extra spots of intramembranous bone formation as well as in intervertebral ligament mineralization were observed. The standard deviation in heart rate did not fluctuate in the zebrafish, according to ultrasound imaging. The ventricular inflow, outflow and myocardial performance index NFT showed a significant difference which might indicate valve abnormalities.
Conclusion: The various findings seem to show that the loss of individual SMAD3 and SMAD6 genes, as well as combinations of deficiency of both, might have an impact on the valve and skeletal abnormalities. However, additional tests are needed to confirm the findings.
Abstract advanced bachelor of bioinformatics 2020-2021 (1): DETECTION OF MICROSATELLITE INSTABILITY (MSI) IN NGS DATA OF TUMOR SAMPLES
As the need for better cancer treatment demands a good classification of the tumor, better and easy classification is needed. For this project, the focus lays on the classification of the microsatellite status of tumors starting from next generation sequencing (NGS) data. The microsatellite status refers to the possibility of mutations in said microsatellites.
With a microsatellite, a small monotone nucleotide repeat (mono-, di-, tri-, tetra-, penta- repeats) is meant. These microsatellites are spread out over the entire genome and is represented by the repeating nucleotide(s), followed by the number of repeats (e.g. “G(10)”). Microsatellites are vulnerable to deficiencies in the DNA mismatch repair (dMMR), this means that during the replication, errors will be implemented into these microsatellites by which the length of the microsatellites will be changed. The most common cause of dMMR is by hypermethylation of the MLH1 promotor. Another cause can by hereditary mutations in MMR genes (MLH1, MSH2, MSH6, PMS2). Mutations in these genes will lead to a disease called Lynch syndrome which increases the chance of developing colon cancer.
It is already proven that there is a link between the microsatellite status and the response to certain immunotherapies. To better be able to detect and treat Lynch syndrome, it is important to detect mutations in microsatellites. If a mutations are present in a number of microsatellites, the tumor will be labeled as having microsatellite instability (MSI), if not, the tumor will be labeled as microsatellite stable (MSS). For now, the main methods used to detect MSI are immunohistochemistry (IHC) and PCR with IHC being the standard protocol for newly diagnosed tumors. As IHC is a very labor intensive test, new methods are being tested. One of these tests is the detection of MSI using NGS data.
By searching through available studies, a tool called mSINGS is seen as a potential tool to detect MSIs using NGS data. mSINGS makes use of a baseline, constructed by using a panel with different loci of microsatellites and MSS samples, to check the status in other samples. Different studies have already shown good results for MSI detection in colon carcinomas. Therefor, the main goal of this internship will be to setup and test mSINGS using sequencing data of tumors from patients of the UZ Ghent.
Different baselines were created using only colon samples or a combination of multiple tumor types. After selecting the best baseline, tests were run to check if the tool was able to correctly determine the status of the tumors. Highly encouraging results for not only colon tumors, but also endometrium, pancreas and gut tumors were found when testing the tool. An official validation is still necessary and will be conducted by Dr. Van der Meulen. Because this tool needs to be implemented into an existing analysis pipeline, scripts were written for different parts of the analysis: pre-processing the data, running mSINGS, parsing the output and cleaning up after the analysis. These different scripts will then be combined into one final script, customized for a specific sequencing run number.
As output for the scirpt an excel is generated. In this file, an adjusted version of the output of mSINGS is given where the loci with instability or a low read depth are highlighted. On a second sheet, a graph is shown where the msings-score for each sample and the threshold line is plotted with a corresponding color for the predicted status of the sample.
Abstract advanced bachelor of bioinformatics 2020-2021 (2): INTERACTIVE VISUALIZATION OF CLINICAL STRUCTURAL VARIANTS IN THE HUMAN GENOME BY A CIRCOS PLOT, IMPLEMENTED WITH THE D3 LIBRARY IN JAVASCRIPT. DATA STORED AND RETRIEVED WITH MONGODB DATABASE
During the last couple of years genetic diagnostics has risen in popularity because of its importance in the medical world. Due to this rise in popularity there is an increasing need for efficient and intuitive visualizations of the complex data received from the field. (Yokoyama & Kasahara, 2019)
Due to the large amounts of complex and often confidential data, a web application is an ideal option for the visualization. A web application is split up into a backend and a frontend. The frontend contains the visualizations and the ability to ask data from the backend. The backend is located on a secure server, where all the data will be stored. This way the data is safe in one database and this makes sure the users can only see the data they are supposed to see.
After reading the article about visualization of structural variants, we decided to go for a Circos plot. This is a circular visualization of the genetic data, where the interactions between the different chromosomes can be easily visualized. This plot is used to get a global view of the data, which makes it useful to easily pinpoint the location of the problem. If the location is known, a more detailed visualization can be used to have a closer look.
In the frontend, the Circos plot is created using D3, which is designed to create interactive plots on the web. This interactivity makes it easier to navigate through the complex genetic data visualized in the Circos plot. The frontend also creates requests for data using JQuery and sends these to the backend, which is a Node.js server connected with a MongoDB database.
The Circos plot consists of multiple tracks of which the order can be changed (with exception of the Translocations track). The Giemsa Stains track shows the Giemsa stains combined with a color code for each chromosome, which is used to differentiate the different locations in the genome. The CNV Analysis tracks shows the copy number variation data, which is useful to check deletions and duplications in the patient. The Big Structural Variants track shows a layered view of all structural variants which are bigger than 1 million base pairs. The Small Structural Variants track shows a heatmap representation of the density of structural variants smaller than 1 million base pairs in a range of 5 million base pairs. And lastly, the Translocations tracks shows all the translocations. The last three tracks are used to visualize the structural variants requested.
The search bar in the user interface can be used to select a certain disease of which the structural variants will be shown after pressing the submit button. There also is an option to choose which chromosomes are shown..
Abstract advanced bachelor of bioinformatics 2019-2020 (1): Automating skeletal and cardiovascular phenotyping of zebrafish
Zebrafish are increasingly used as a versatile animal model which can be used to efficiently and rapidly model genetic disorders. An advantage of working with zebrafish during the early stages of development is that large numbers of specimens can be examined in a short time frame, enabling the design of in vivo high-throughput screening programs. At the Center for Medical Genetics Ghent, several zebrafish models of heritable connective tissue disease have been generated which show skeletal and cardiovascular phenotypes. the high amount of data generated and collected during these screens inevitably leads to a bottleneck in data analysis and interpretation. Automated processes are necessary to handle these large volumes of complex data in an efficient and unbiased manner. The Jupyter Notebook is a useful tool for speeding up the workflow, and interactively developing and presenting data. In this project the focus was on the unbiased analysis of Transmission Electron Microscope (TEM) images containing areas of collagen fibrils, which are an important building block of connective tissue. Based on a series of TEM images from zebrafish skin, a first pattern recognition script was developed. Certain parameters such as collagen fibril diameter, fibril count and occupied area percentage can be automatically detected and used to determine differences between controls and zebrafish disease models. This is accomplished using Laplacian of Gaussian Blob Detection, a method using convolution in order to detect points in a two-dimensional image that differ in properties compared to the surrounding regions. While the first script can be used to automatically analyze a large amount of images consecutively, some samples require manual evaluation in order to filter out irregularities such as fibroblasts, cell nuclei and technical artefacts which are also detected by the automated script. With the second script it is possible to filter out these spots that were incorrectly detected, resulting in more precise calculations. Currently these incorrectly selected spots cannot be excluded automatically, due to the strong similarity to the actual collagen fibrils. In the future it might perhaps be possible to implement an extra filter using a machine learning approach to improve the accuracy of the automated selection algorithm.
Abstract advanced bachelor of bioinformatics 2019-2020 (2): Combining 3DGenomics with epigenomics to discover novel biomarkers in T cell leukemia
T-cell acute lymphoblastic leukemia (T-ALL) is an aggressive type of leukemia that progresses quickly. The survival rates have been improving over the years, however, the prognosis upon disease recurrence remains dismal. An increasing number of studies have already shown the importance of epigenetic deregulation in T-ALL development. The goal of this research is to explore the epigenetic differences between the leukemic cells and their normal T-cell counterparts, that show correlation with refractory disease.
To profile the epigenetic landscape of T-ALL, ChIPmentation of the histone marks H3K27Me3, H3K27Ac and H3K4Me3 on 6 T-ALL and 11 normal T cell samples was analyzed. The raw data consists of files in FASTQ format, more specific: single end reads of 75 base pairs. Input data corresponding to the immunoprecipitated samples serve as a control to more reliably determine the presence of these histone marks.
To analyze the raw data, a pipeline was constructed. First, the files were merged per sample. To assure the quality of the data, a quality control was performed with the tool “FastQC”. These results showed high adapter content and therefore the samples were trimmed with the tool “Trimmomatic” in order to remove the adapters and to only keep the high quality part of the sequence. The results of the trimmed files were again evaluated with “FastQC” and this showed that the adapter content dropped significantly while only a small percentage of reads was lost during the process. Next, the trimmed files were aligned to the human genome (hg38) with the tool Bowtie2. After the alignment, the SAM files needed to be converted to sorted BAM files since the following steps required this format. Subsequently, peak-calling was performed on the sorted BAM files with “MACS2” and the coverage was calculated with “deepTools”.
After testing the separate scripts on one histone mark, the scripts were merged into a concise one-script pipeline. Afterwards, this pipeline was applied to process the data for the other histone marks. The advantages of this pre-processing pipeline are that it is faster, less prone to errors and can be executed on different systems because the versions of the tools are specified.
Finally, a differential analysis was performed on the processed data. First, a count matrix was constructed in R from the sorted BAM files with the unique peaks as a reference. Subsequently, normalization was performed on the dataset constructed from this count matrix. The variance in the dataset was assessed by principal component analysis and by hierarchical clustering of highly variant sites. Significantly different binding sites were annotated to their closest gene and plotted in a heatmap. We could conclude that clear differences between leukemic cells and their normal counterparts are visible in both the PCA plot and the heatmaps. Since the differential occupancy of histone marks on presumably important regulatory sites can now be evaluated with high reliability, we aim to integrate this dataset with information on the 3D structure of the genome in order to be able to identify a set of potential epigenetic biomarkers in T-ALL.
In conclusion, a pipeline was constructed successfully and can also be applied for future ChIP-seq and ChIPmentation data. Furthermore, a clear distinction could be made between the normal T cell profiles and T-ALL. These results are promising and integration of data covering the 3D conformation of the human genome in both T-ALL and normal T cells might unravel novel molecular mechanisms driving T-ALL, which could not yet be performed due to time limitations. Thus, further research is required to discover novel biomarkers that might improve the treatment stratification of T-ALL in the clinic.
Abstract Bachelor Project FBT 2019-2020: The impact of Olaparib on BRCAwt ovarian cancer cell lines SKOV3 and A2780 tested in vitro and in vivo with the zebrafish xenograft
Epithelial ovarian cancer are normally treated with the standard treatment, which is a primary debulking surgery and chemotherapy. This therapy is not effective for each patient, which creates a need for a targeted therapy. The PARP inhibitor Olaparib is currently used as a targeted therapy for BRCA mutated ovarian tumors. This study investigates whether the effect of Olaparib also has an inhibitory effect on BRCA wild type ovarian cancers.
By testing BRCA wild type ovarian cancer cell lines A2780 and SKOV3 in vitro with an Olaparib treatment, the inhibition coefficient of Olaparib in these cell lines can be determined. With the results of the in vitro assay, cell lines will be tested in vivo in a zebrafish xenograft.
The in vitro assay of A2780 cells has shown that Olaparib has an inhibitory effect on cell growth, but the inhibition coefficient is not reached with this setup. After this assay, the experimental part of the study has been halted. An exploratory study of different methods for determining the inhibition coefficient of the cell lines and a comparison of different manners to make a zebrafish xenograft has been added to the study.
In the future, the in vitro study must be continued on both cell lines before the in vivo treatment can be started. Possibly a technique based on caspase enzymes can be used for the in vitro study.
Abstract Bachelor Project FBT 2018-2019: In vitro evaluation of new treatments in ovarian cancer cell lines
Ovarian cancer is the seventh most commonly diagnosed cancer among women in the world and unfortunately associated with bad prognosis. Despite high initial response to currently used therapies, most patients relapse and develop chemoresistance. Alternative molecular based specific therapies for patients with ovarian cancer are urgently needed to improve clinical outcomes and the quality of life.
The aim of this study is to evaluate the cytotoxic effects of both bromodomain and extra- terminal motif inhibitor (BETi) and MAPK/ERK-kinase inhibitor (MEKi) and their relation to an activated mitogen-activated protein kinase pathway (MAPKp) in ovarian cancer cell lines. First, the KRAS mutation c.35G > T was evaluated in several cell lines by Sanger sequencing.
Secondly, both single and combination therapies of a BETi (1 µM) and MEKi (1 µM) were evaluated on the following ovarian cancer cell lines: ES-2, M28/2, SKOV-3 and A2780 by in vitro plate based assays. The cytotoxicity was evaluated in three ways: 1) Crystal violet staining is a cheap and indirect method to quantify possible cytotoxicity by measuring the cell viability, 2) Apoptosis was evaluated by a luminescent assay, called Caspase-Glo® 3/7 that determinates caspase-3 en -7 activity, 3) Proliferation was observed using IncuCyte®, with subjective masking.
The KRAS mutation c.35G > T was detected in the M28/2 cell line which leads to a protein modification resulting in an ATP-independent MAPKp activation. Hypothetically, there is more apoptosis in cells treated with BETi which has cytostatic effects compared to untreated cells. More apoptosis is expected in M28/2 in the test condition MEKi in comparison to BETi because MEKi induces direct apoptosis in the MAPKp activated cells resulting in a higher cytotoxicity compared to BETi. In the non-mutated cell lines (A2780 and SKOV-3), the difference between BETi and MEKi depends on the degree of MAPKp activation. A synergy has to be observed in the wild-type cell lines because of the double blockade of MEK and BET pathway and increased activation of MAPKp induced by JQ1. More apoptosis is expected in the test conditions MEKi and MEKi + BETi in the M28/2 in comparison to a non-mutated cell line, due to the constitutive activation of the MAPKp. Literature reported that ES-2 has a BRAF-mutation which causes increased sensitivity for MEKi. There is more information needed about the effect on the MAPKp to make a hypothesis about the difference between MEKi and BETi, but there is definitely a synergy expected because of the double BET and MEK blockade.
The crystal violet staining reveals that the viability is significant higher in the untreated cells compared to the test conditions containing MEKi, BETi or a combination of both (p – value < 0,05). Between MEKi, BETi or MEKi + BETi there is no significant difference found for the M28/2 and A2780 cell line (p-value > 0,05).
The same trends were obtained by the caspasetest showing more apoptosis in the test condition with the inhibitors compared to untreated cells. In contrast to the crystal violet assay, caspase3/7 showed significant differences between MEKi, BETi and MEKi + BETi for the M28/2 and ES-2 cell line (p-value < 0,05). For the M28/2 cell line there is more apoptosis measured in the test condition MEKi in comparision to BETi confirming the hypothesis. Furthermore cytotoxic synergy is observed in both cell lines when BETi and MEKi are combined.
The SKOV-3 cell line gives the most expected results for the IncuCyte® experiment; the cell proliferation is inhibited in the test condition containing MEKi or BETi with a noticeable synergy seen when both inhibitors are combined.
In future studies more reliable results can be generated by improving the standard deviations and repeatibility. Therefore, critical steps such as seeding of cells and application/removal of fluids from wells need standardization. Eventually the wash steps during crystal violet staining can be optimized. In addition to that, more technical and real repeats are needed to assure the reproducibility of the assays.
Abstract advanced bachelor of bioinformatics (1) 2018-2019: Benchmarking of protein coding potential prediction algorithms on small ORF datasets
Protein coding prediction algorithms are tools that predict the coding potential of protein sequences. Most of these prediction algorithms work on and are benchmarked on long open reading frames (ORF; ≥ 300 nucleotides). The aim of this research is to compare a selection of such algorithms and benchmark them on small open reading frames (sORF; <300 nucleotides). The selected algorithms were CPAT, PLEK and PORTRAIT. First, four different sORF datasets were obtained from the sORF.org website, an online collection of known sORFs based primarily on ribosome profiling studies. These four datasets comprise either all sORFs or subsets based on conservation and whether or not the sORFs were reported in the landmark Bazzini et al,2012. After the analysis with the three different prediction tools, CPAT and PORTRAIT had similar results. With CPAT a specificity was obtained ranging from 23.80% (highly conserved sORFs reported by Bazzini et al) to 44% (full set) and for PORTRAIT slightly higher specificities were found (between 31,74% and 48%). PLEK could not predict any of the protein coding small ORFs and as such had a specificity of 0% for all four datasets. To study the effect of the size of the ORF on the predictions, a second benchmarking approach was made by creating positive and negative datasets in silico. The positive sets are made by truncating sequences of known protein coding genes. Three positive sets were created, with a length of 150, 300 and 450 nucleotides (nt), followed by a stopcodon. For the negative sets, known non-coding sequences were used, adding a start and stopcodon at the beginning and end of the 150, 300 and 450 nt sequences. After the analysis, CPAT had a specificity ranging from 32.29% (150 nt) to 94.33% (450nt) and a sensitivity ranging from 95.52% (450nt) to 99.25% (50nt). This implying the specificity increases, and sensitivity decreases over the nucleotide length. PORTRAIT only obtained results for sequences longer 160 nucleotides, with a specificity ranging from 76.58% (300 nt) to 94.33% (450 nt) and a sensitivity of 100% (150 nt) to 83.80% (450 nt). Indicating the same trend as CPAT, with a increasing specificity and decreasing sensitivity over sequence length. PLEK had a specificity of 0% for the 150 and 300 nt sets. This moved up slowly with a 2.20% specificity for the 450 nt sequences. It did record the highest sensitivity ranging from 99.98% (450 nt) to 100% (150 nt and 300 nt). This research shows that none of the selected algorithms recorded a high specificity for detecting the coding potential for small ORFs lower than 160 sequences. For small ORFs longer than 160 nucleotides CPAT and PORTRAIT are both reliable algorithms, keeping in mind that PORTRAIT has a lower sensitivity. PLEK, however, is not advised to predict the coding potential of small ORF, not being able to predict any protein coding small ORF. Further studies are necessary to analyze the existing algorithms to what cause this bias for long ORF, and how to create accurate, and therefore reliable algorithms for the prediction of small ORF.
Abstract advanced bachelor of bioinformatics (2) 2018-2019: Decoding plasma RNA profiles
Is it possible to use circular RNA (circRNA) as a cancer biomarker? To answer this question, a pilot project was started where circRNA data from plasma pools (1 pool per cancer type) was collected in the Center for Medical Genetics Ghent. To proceed with the research and discovery of these potential biomarkers for cancers, the database will be extended with more samples in the near future. However, in this project the comparison was made between circRNA profiles in these plasma pools and in the tissue of origin of the cancer. The publicly available data from MiOncoCirc will be used for the comparison with the inhouse data, this dataset contains 2000+ cancer tissue samples across 40 cancer types (Josh N. Vo, 2019) (The University of Michigan, sd). To compare the MiOncoCirc with the inhouse data, it needs to be adapted to a useable format by making use of the R programming language. The data will also be visualized to get an overview of how the data is formatted and to select the data that will be useable for the project. As a start to the project the MiOncoCirc data was adapted to a more useable format. This was accomplished by firstly cleaning up the data to make sure the data is in the same format as the inhouse plasma data. In order to do so, all cancer type annotations were matched, data was capitalized and spaces were replaced by underscores. In the original data no identifier was given for the different circRNAs so these were created by merging the chromosome name with the start and end position on the chromosome. Because of the size of the dataset there where some memory issues when a count matrix was constructed. As a solution, a more compact matrix was created by making use of the min, max, mean or median of the counts. The data exploration part of the project consisted out of General statistics: Number of samples for each cancer type, distribution of gender for the cancer types, are some more common in female/male? Adaptation of existing scripts for the calculation of fold change and specificity. Shiny app for visualization of circRNA counts and showing the data from fold change/specificity calculations. To visualize the relationship between the two datasets, Venn diagrams were used. These Venn-diagrams visualize the common circRNAs for each cancer type in relation to each other. From the visualized relation between the two datasets a conclusion can be made that the data from MiOncoCirc is not viable to include in the inhouse dataset at this moment for this specific project, because of the minimal overlap between the 2 data sets. To exclude the possibility that this is a side-effect of different preprocessing, the raw data from MiOncoCirc will be rerun with the inhouse pipeline to make sure the preprocessing of the data is done in the same way.
References
Josh N. Vo, M. C. (2019). The Landscape of Circular RNA in Cancer. The University of Michigan. (sd). mioncocirc. Found at https://mioncocirc.github.io/.
Abstract advanced bachelor of bioinformatics (3) 2018-2019: Genomics data management for analysis and visualization tools
The project is part of the genomics data management platform, a platform used in a flexible analysis environment for pipelines and visualizations among others. An important aspect to keep in mind for this data management are the FAIR principles. FAIR stands for Findable, Accessible, Interoperable and Reusable and is embraced by the Global Alliance for Genomics and Health (GA4GH). It represents the idea to have a general groundwork for data-sharing infrastructures. If these principles are followed, all researchers, clinicians, pipelines,… know that the data can be obtained in a clear, standardized way. Before starting on the main code for the platform, a basic understanding of the MinIO servers was needed. MinIO is an implementation that offers object storage conform with the Amazon Web Service (AWS) S3 REST API. With S3 standing for Simple Storage Service and REST API meaning Representational State Transfer Application Programming Interface. The server is made to store unstructured data, called objects, that can be organized in clearly labelled buckets. The primary work of the project was done on an API codebase for the Data Repository Service (DRS). The DRS API provides a generic interface so a user or workflow can access the data in a standardized way. This is done by using a logical identifier to retrieve the data it represents. The ID itself however has some guidelines. It needs to be URL-safe, always link to the same object and there may be more than one ID per object. The DRS API was split up into multiple scripts to keep a clear overview of everything and to make sure no code repetition was done. The API is started with the main.py script. All routes from the get statements that fetch the needed data, are grouped in the api.py script before being imported in the main file. There are four get statements used in the DRS API. The first one is the GET /bundles/{bundle_id} that returns the bundle metadata and a list of ids that are used to obtain the bundle contents. GET /objects/{object_id} returns in its turn the object metadata and a list of the access methods for the retrieval of the object bytes. The GET /objects/{object_id}/access/ {access_id} goes further on the previous one. It will return a URL that links to the MINIO server in order to collect the object. this will only be called if an access_id is given. An example of when this is the case, is when a server uses a signed URL to collect the object bytes. The last get statement is GET /service-info. It is designed to return the service version and other information. Models were made for the objects, bundles and service-info so that the metadata always gets shown in the same standard way. In order to store the data that link to these models, an SQLite database was made. In this database all the important groups of data from the API models get put in corresponding tables. It is set up in such a way so the right data can be found for each object and/or bundle. The previously mentioned get statements use these models for their output. The end result is to get a link from the DRS to the previously mentioned object storage. This link is a URL that can be optionally secured with an access layer that works with tokens from Oauth2. By making sure that the FAIR principles are kept in mind, users have an easy way to retrieve the desired objects or bundles while the output always remains in the same format. This level of consistency is crucial in a professional work environment in order to maintain a good flowing data sharing system.
Abstract traineeship advanced bachelor of bioinformatics 1 2017-2018: Exploratory data analysis on Total RNA Sequencing from COPD patients
Introduction: Chronic Obstructive Pulmonary Disease (COPD) is a life-threatening pulmonary disease characterized by a persistent airflow limitation and destruction of alveolar walls (=emphysema), whose pathobiology is not completely understood. COPD results from a complex interplay between genetic susceptibility and environmental exposure, most importantly tobacco smoke. Nevertheless, it is estimated that only 15-20% of smokers develop COPD, suggesting that underlying (epi)genetic mechanisms could be involved. The goal of this study is to identify a set of non-coding and protein-coding RNAs which are dysregulated in lung tissue of patients with COPD using total RNA sequencing.
Methods: RNA was extracted from lung tissue of 32 patients, encompassing 10 never smokers, 9 smokers without airflow limitation and 13 smokers with COPD. Total RNA sequencing was performed with an Illumina HiSeq 4000 Sequencing System on paired-end TruSeq RNASeq libraries. The sequencing reads were aligned to the reference human transcriptome using 3 different tools, namely STAR, BWA-MEM and Bowtie2, after which HTSeq was used to quantify transcript expression. A filtering step was performed to remove genes with zero or low counts. Next, statistical analyses were performed using the statistical programming language R (v 3.5.0) and the R packages Limma, edgeR or DESeq2. Differentially expressed (DE) genes between 2 studied groups were based on an adjusted p-value < 0.05 and a log2-fold change > 1. Finally, Gene Set Enrichment Analysis (GSEA) was carried out with the javaGSEA desktop application using Gene Ontology – Biological Process (GO-BP) and Kyoto Encyclopedia of Genes and Genomes (KEGG) gene sets (downloaded from the Molecular Signatures Database v4.0, Broad Institute). A comprehensive overview of the RNA seq analysis workflow is demonstrated in Figure 1.
Results: Exploratory analysis showed that the read count distribution was highly variable between the samples, after which we removed the samples where the reads were below 75 million, ending up with a total of 21 samples (6 never smokers, 7 smokers and 8 patients with COPD). 22109, 22344 and 20845 genes were detected as expressed with respectively STAR, BWA-MEM and Bowtie2 after filtering. DE analysis found only 2 genes or less (depending on which R package was used) to be significantly upregulated in smokers versus never smokers and 4 (or less) to be upregulated in patients with COPD compared to never smokers. No genes were DE between smokers with and without COPD. Importantly, the 3 software packages (Limma, DESeq2 and edgeR) identified the same DE genes. GSEA pointed towards positive enrichment of pathways such as inflammatory response, innate immune response, regulation of cytokine production, in smokers and patients with COPD compared to never smokers.
Conclusion: Total RNA sequencing analysis on lung tissue of never smokers, smokers and patients with COPD detected only a minority of genes to be differentially expressed between diseased and healthy subjects, whereas GSEA pointed towards an upregulation of pathways associated with inflammation/immunity in smokers with or without COPD.
Abstract traineeship advanced bachelor of bioinformatics 2 2017-2018: DNA copy number analysis using RNAseq data
Cancer genomes are characterized by DNA copy number changes, which are typically measured at the DNA level. Here, we evaluated the possibility to infer DNA copy number changes from RNA-seq data, based on measuring the ratio of expression between the two alleles.
The main advantage is that RNA can be used for different research purposes. It would be useful to add detecting aberrations to the list.
To confirm the self-made pipeline is correct, we compared results with copy number profiles derived from matching DNA samples.
The package ‘Changepoint’ is introduced to calculate the changepoints of the Allelic ratio. The penalty method of the function was optimized. If the changepoints are calculated separately for each chromosome, the outcome is more accurate. To avoid false exclusion of important parts, a variable baseline is calculated. A baseline of 15% above the lowest changepoint in combination with ‘Hannan-Quinn’ for penalty and the method ’PELT’ shows the best results.
Subsequently, we compared expression of genes in aberrant regions between the tumor sample and the matching normal sample (if available). The tumor sample and the mean of all the normal samples comparison is below and the last new comparison is the matching tumor versus the mean of all the tumor samples.
To make the third graphic more clear, a new script is written. It compiles a CSV-file which contains the genes that occur and doesn’t occur in the wanted regions for all samples of the same cancer type. The main script will only take the tumor samples where there isn’t an aberration (those who don’t occur in the wanted region) in the region to calculate the mean. Thus the tumor sample of interest is compared with the mean expression of the tumors where there is no aberration in that region.
The figure shows the output of the script. All graphs have the same layout: the full vertical lines indicate the end/beginning of the chromosomes, striped lines locate the centromeres.
The graph on the top shows the allelic ratio in red. The lines in blue are the regions determined by the changepoint package. The calculated baseline is the horizontal line. All regions above the line are the regions that are going to be looked at more closely in the next graphs.
The purpose of the CNV graph is to compare the self-made algorithm with the results derived from the matching DNA. When the line is clearly above the zero a gain is called, while below is a loss.
The third graph shows the comparison between the matching normal sample of the same person and the tumor sample. Below is the comparison of all gene points of the matching tumor versus the mean of the normal samples. The last graph is the tumor sample of interest compared to mean of the tumor samples without aberrations in the specific regions.
To conclude, the pipeline needs to be tested on multiple samples of multiple cancer types to determine its accuracy and points that need to be optimized. As yet today, the pipeline is correct in most of the cases.
Abstract traineeship advanced bachelor of bioinformatics 3 2017-2018: Creating a responsive interface for long non-coding RNA database LNCipedia
LNCipedia, https://lncipedia.org, is a database for human long non-coding RNA (lncRNA) transcripts and genes. LncRNAs constitute a large and diverse class of non-coding RNA genes.
The database is publicly available and allows users to query and download lncRNA sequences and metadata based on different search criteria. The database may serve as a source of information on individual lncRNAs or as a starting point for large-scale studies.
LNCipedia is built using Mojolicious, a web framework for the Perl programming language based on the MVC (Model View Control) pattern.
The current interface of LNCipedia is however not responsive or mobile friendly. Therefore, the main goal of this project was to redesign the website as a mobile first web application.
Bootstrap's CSS and JavaScript libraries were used to create the design. Docker was used to build and run the app locally. GitHub was used for version control.
Additionally, a python tool was created to add meta-information to the LNCipedia database. More specifically, the tool determines if the human lncRNA transcripts are conserved in other species.
Abstract traineeship advanced bachelor of bioinformatics 4 2017-2018: Development of a new variant classification tool based on Sherloc classification criteria for variants in Mendelian diseases
The American College of Medical Genetics and Genomics-Association for Molecular Pathology (ACMG-AMP) guidelines are used as a common framework for variant classification. However these guidelines lack specificity, are subject to varied interpretations, or fail to capture relevant aspects of clinical molecular genetics. Implementation of the current guidelines has been shown insufficient for a good variant classification.
The “Centrum Medische Genetica Gent” (CMGG) uses these ACMG-AMP guidelines to classify variants from hereditary diseases. However a refinement of the variant classification criteria is needed. This is done by implementing a new classification model based on Sherloc classification criteria (Nykamp et al, 2017).
Sherloc builds on the framework of the established ACMG-AMP guidelines and makes 108 refinements to it, which makes it a more consistent and transparent variant classification tool. It is based on a weighting system. It uses a semiquantitative system in which each criterion is awarded a preset number of points on benign or pathogenic scales (1B-5B or 1P-5P), which reflect the value of the data type toward the overall classification argument (Figure 1a). Accumulated benign and pathogenic evidence types are summed separately and compared against preset thresholds. Eventually classifying the variant in one of the five groups; benign, likely benign, variants of uncertain significance, likely pathogenic and pathogenic. There are five evidence categories (Figure 1b) divided in two groups which contribute to the final score.
Clinical criteria:
- Population data
- Clinical observations
Functional criteria:
- Variant affect
- Experimental studies
- Computational & Predictive
The goal of this project is to design and implement a new web tool, which uses the refined ACMG-AMP guidelines and is more detailed/precise than the previous one.
The 108 Sherloc classification criteria and underlying results were successfully implemented in a web based tool written in HTML/PHP. The tool consists of a series of interactive and dynamic decision trees guiding the user to the selection of benign or pathogenic evidence types. Depending on which evidence types are checked, the variant gets classified in a certain class. The submitted evidence types and final variant class are reported afterwards.
Abstract bachelorproef 2017-2018: Molecular characterization of MGMT promotor methylation and IDH1, IDH2 and BRAF mutations in patients diagnosed with glioblastoma
Wegens confidentialiteit kan de samenvatting niet gepubliceerd worden.
Abstract traineeship (advanced bachelor of bioinformatics) 1 2016-2017: Targeted mutation detection in patients with Hereditary Hemochromatosis and MTHFR deficiency
The main aim of the research was to detect specific mutations that cause the diseases hereditary hemochromatosis and homocystinuria due to MTHFR deficiency. Hereditary hemochromatosis is a clinical disorder with bad regulation of iron in the body, which can cause (severe) damage to the organs. This disease can be caused by three specific substitutions in the HFE gene: c.845G>A (p.Cys282Tyr), c.197G>C (p.His63Asp) and c.193A>T (p.Ser65Cys). The most important mutation of these three is c.845G>A. Up to 90% (40-90%) of the clinical cases of hemochromatosis have this mutation. Homocystinuria is a disease where the amino acid homocysteine is increased in the body. This disease can be caused by a rare mutation in the MTHFR gene which leads to MTHFR deficiency: c.665C>T (p.Ala222Val). The enzyme made by MTHFR play a role in converting homocysteine to methionine.
Before the research, these mutations were detected using a Lightscanner® system. Because support was no longer available for this instrument, a new way to detect these mutations was necessary. Polymerase Chain Reaction (PCR) combined with Next Generation Sequencing (NGS) could be the solution. However, preliminary results with this new way of mutation detection showed a detection of too many false positive variants. To prevent unwanted mutations being reported, the goal was to write a Python script which examines and reports only these four mutations of interest.
The process starts with a PCR reaction on, from blood extracted, DNA from selected patients. For the amplification of the region of interest in the HFE gene, two PCR reactions are needed, for the amplification of the MTHFR region of interest only one PCR reaction is sufficient. The PCR products are then handed over to a specific ‘MiSeq team’. NGS is performed by this team on a MiSeq instrument (Illumina sequencing technology). The result of the sequencing process are .bcl files. These are converted to multiple fastq files. The next step is a quality control (quality trimming) on these files; bad quality ends are removed from the sequences. The final step is the mapping against the human reference genome (Hg19). After the mapping, five files are obtained: a coverage file, a variant track file (the most important one), a mapping file, a structural variants (SV) file and an indel file (insertions and deletions).
For each MiSeq run, a runinfo file is created. In this file, patients from the run are listed with the tested gene or gene panel. Only the patients with HFE and/or MTHFR as tested gene will be evaluated by the script.
The script reads the coverage and variant track files for each HFE/MTHFR patient in the runinfo file. All variants in the variant track file are compared with the three mutations from hemochromatosis and the mutation from homocystinuria. When a mutation is found in a patients file, following information about the mutation in the patient is captured from the variant track file and coverage file: c-notation, p-notation, status (heterozygote, homozygote, wild type, Sanger), the used PCR assay and the exon number. As output file, an Excel file is generated per patient per gene. In this file, all of the captured information is written. The script also creates an overview file which summarize all this information for all patients.
The written script was successfully tested and can now be used to analyze new MiSeq runs with HFE and/or MTHFR patients.
Abstract traineeship (advanced bachelor of bioinformatics) 2 2016-2017: Development of an RNA-seq pipeline to determine DNA copynumber status
RNA sequencing (RNA-seq) is considered a powerful tool for gene expression analysis and gene discovery. Because of its nucleotide resolution, RNA-seq can also be applied to identify variants and quantify allelic expression levels. As allelic expression levels are, in part, driven by the copy number of the individual alleles, imbalances in allelic expression ratio’s may point to changes in DNA copy numbers.
Most cancer cells are characterized by DNA copy number changes that result in the gain or loss of alleles. The chromosomal regions that are subject to these changes often harbour important oncogenes or tumour suppressor genes and can be highly characteristic for individual cancer types. Typically, these changes are quantified at the DNA-level, using shallow whole genome sequencing or array CGH (Comparative genomic hybridization). With this project, we aimed to evaluate the use of allelic expression ratio’s, derived from RNA-seq data, to map genome-wide copy number variations in cancer cells. The performance of our approach was assessed by direct comparison with matching DNA copy number data (determined using aCGH).
Regions with an allelic ratio of more than 1,75 in the RNA-seq data were marked as regions with ‘allelic imbalance’. These regions nicely coincided with gains or losses that were called based on the available DNA data. To be able to determine if the allelic imbalance was caused by a gain or a loss, we investigate the genes within the region of allelic imbalance and compare their expression in the tumour sample to a matching normal sample or to the mean of all the normal samples when there is no match. The overall tumour to normal ratio of genes within the region are applied to distinguish gains from losses.
The allelic imbalances are on the same position as the gains and losses shown by DNA copy number data. When there is more expression in tumour, there is a gain and a ratio of more than 0 on the bottom line. If there is less expression in tumour, there is a loss and a ratio of less than 0.
To be able to get DNA copy number information directly from RNA-seq data is a huge advantage as this would allow us to investigate both gene expression, variants and DNA copy number status from a single dataset without the need for DNA analysis.
Abstract bachelorproef 2016-2017: Optimalisatie en validatie van een NGS approach voor mutatiedetectie in HFE en MTHFR en bepalen van de prevalentie van varianten in geselecteerde extragenische regio’s
Wegens confidentialiteit kan de samenvatting niet gepubliceerd worden.
Abstract bachelorproef 2015-2016: Molecular analysis of RECQL and cancer susceptibility genes in families with a strong predisposition to breast and ovarian cancer
Wegens confidentialiteit kan de samenvatting niet gepubliceerd worden.
Address
De Pintelaan 185 C. Heymanslaan 10
9000 Gent
Belgium |
Contacts
Jo Vandesompele (BIT) Jan Hellemans (BIT) Bram De Wilde (BIT) Tom Sante (BIT) Jasper Anckaert (BIT) Pieter-Jan Volders (BIT) Björn Menten (BIT) Toon Rosseel (BIT) Francisco Avila Cobos (BIT) Annelien Morlion (BIT)
09/3323603 (BIT) |
Kathleen Claes (FBT) Kim De Leeneer (FBT) Joni Van der Meulen (FBT) Juliette Roels (FBT) Charlotte Fieuws (FBT) Patrick Sips (FBT)
09/3322478 (FBT) |