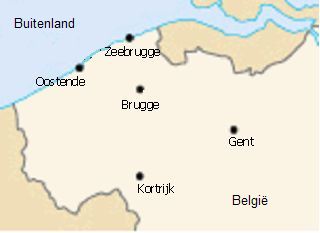
Pages
VIB – Bioinformatics Core (BITS)
A lot of datasets on protein-protein interactions (PPI) are publicly available at this moment. These PPI datasets are spread across multiple data sources. Naively combined, the newly created data source may contain a lot of interaction pairs which appear different due to the current annotation of the datasets but are in fact identical. Some PPIs are unique to a single source.
The goal of the iRefIndex project is to make a unifying interaction index to bring all this interaction data together. Redundant interactions and objects aka protein interactors are brought together in groups called ‘the redundant interaction groups’ or RIGs and ‘the redundant object groups’ or ROGs. This way, duplicated proteins or protein interactions are avoided in the index.
To set up this index, a sophisticated pipeline has been created by Donaldson et al eight1 years ago. By providing a configuration file, it is possible to automatically download the preferred source files from the internet or use a local source. In this case, 24 sources including MatrixDB2, Reactome3 and HPIDB4 were used to obtain the necessary interaction data. These source files can be in mitab format, XML format or have their own specific format. In addition, 9 curation sources are used, such as Entrez-gene5 and RefSeq6. These sources all have their own specific structure. After the download the files are parsed in the coherent format. For the mitab format, this means the PubMed-ID’s, the authors, the aliases, interactions, etc. are all described in separate files now. After the parsing, these separate files are imported into the iRefIndex database. The last step is building the index from this database. The result of this pipeline is a mitab file containing all interactions and interaction partners.
The goal of this project was to optimize the pipeline used to create the iRefIndex version 17. The main problem is that some files are malformed or even have changed format, for example changed from an XML-format to a mitab-format or removed an entire column in the source file. These malformations or changes need correction to further process them. These corrections need to be made either in the source file or in the parsing scripts. If there is a malformation in the source file, after the final release, we aim to report to the maintainer of this source.
In the final version of the iRefIndex version 17, four new sources were added in comparison with version 16: BAR7, MINT8, HuRI9 and SPIKE10. The MOLCON source is not included since the source was not available anymore.
Another difference to the iRefIndex version 16 is that not only interactions reported with a PubMed identifier are included into the index, but also the ones with a DOI identifier. By adding sources, the iRefIndex gets enriched, since new unique interactions are added to it. For example, the HuRI source has 51482 different interactions, from which 10368 are unique to the HuRI source.
In total, 153527 unique proteins and 1185907 unique protein interactions or complexes are available in total in version 17. This is an increase in comparison with version 16, which contained 135893 unique proteins and 967265 unique protein interactions or complexes.
Sources
- Razick, S., Magklaras, G. & Donaldson, I.M. iRefIndex: A consolidated protein interaction database with provenance. BMC Bioinformatics 9, 405 (2008). https://doi.org/10.1186/1471-2105-9-405
- Launay, G., Salza, R., Multedo, D., Thierry-Mieg, N. and Ricard-Blum, S. (2015) MatrixDB, the extracellular matrix interaction database: updated content, a new navigator and expanded functionalities. Nucleic acids research, 43, D321-327.
- Fabregat, A., Jupe, S., Matthews, L., Sidiropoulos, K., Gillespie, M., Garapati, P., Haw, R., Jassal, B., Korninger, F., May, B. et al. (2018) The Reactome Pathway Knowledgebase. Nucleic acids research, 46, D649-D655.
- Ammari, M.G., Gresham, C.R., McCarthy, F.M. and Nanduri, B. (2016) HPIDB 2.0: a curated database for host-pathogen interactions. Database: the journal of biological databases and curation, 2016.
- Maglott D, Ostell J, Pruitt KD, Tatusova T. Entrez Gene: gene-centered information at NCBI. Nucleic Acids Res. 2005;33(Database issue):D54‐D58. doi:10.1093/nar/gki031
- Pruitt KD, Tatusova T, Maglott DR. NCBI Reference Sequence (RefSeq): a curated non-redundant sequence database of genomes, transcripts and proteins. Nucleic Acids Res. 2005;33(Database issue):D501‐D504. doi:10.1093/nar/gki025
- Toufighi K, Brady SM, Austin R, Ly E, Provart NJ. The Botany Array Resource: e-Northerns, Expression Angling, and promoter analyses. Plant J. 2005;43(1):153‐163. doi:10.1111/j.1365-313X.2005.02437.x
- Orchard, S., Ammari, M., Aranda, B., Breuza, L., Briganti, L., Broackes-Carter, F., Campbell, N.H., Chavali, G., Chen, C., del-Toro, N. et al. (2014) The MIntAct project--IntAct as a common curation platform for 11 molecular interaction databases. Nucleic acids research, 42, D358-363.
- Luck, K., Kim, D., Lambourne, L. et al. A reference map of the human binary protein interactome. Nature 580, 402–408 (2020). doi:10.1038/s41586-020-2188-x.
- Paz A, Brownstein Z, Ber Y, et al. SPIKE: a database of highly curated human signaling pathways. Nucleic Acids Res. 2011;39(Database issue):D793‐D799. doi:10.1093/nar/gkq1167
The goal of the project is to support the integrative efforts at VIB bioinformatics Core facility, with emphasis on integration of data from VIB’s research activities. In specific, state-of-the-art bioinformatics software are used to integrate proteomics, metabolomics, and transcriptomics data sets. Limitations in the functions of these software are identified and optimized in towards making them more user friendly for biologists who lack or have very basic computational skills, for enhancing biological discoveries.
Rapid technological advances have led to the massive production of different types of biological (big) data and enabled construction of complex networks with various types of interactions between diverse biological entities. Single omics analysis methods were shown to be limited in dealing with such heterogeneous networked data. Integrative methods can collectively mine multiple types of biological data and produce more holistic, systems-level biological insights [1].
Principal component analysis (PCA) combined with sparse Partial Least Squares (sPLS) implemented in the R package mixOmics [2], Weighted Gene Co-expression Network Analysis (WGCNA) [3] and Multi-Omics Factor Analysis (MOFA) [4], are used to summarize and decipher two data sets consisting of transcriptomics, proteomics and metabolomics experiments from Mus musculus and Medicago truncatula. These three robust, statistical approaches, were designed to uncover driver genes that are responsible for numerous cellular processes. While PCA is an appropriate and commonly used method, WGCNA holds several advantages in the analysis of highly multivariate, complex data by modelling them as networks/modules [5].
The WGCNA R software package incorporates functions for performing various aspects of weighted correlation network analysis such as network construction, module detection, gene selection and visualization. MOFA is a factor analysis model for the integration of multi-omic data sets. Once trained, the model output can be used for downstream analyses, including the visualisation of samples in factor space and enrichment analysis. The mixOmics R package proposes several multivariate methods that are suited to large omics data sets and that have the properties of reducing the dimension of the data by using components that are used to produce graphical outputs that shows the relationships and correlation structure between the different integrated omics.
Whereas mixOmics and MOFA R packages can be run easily, WGCNA contains a few functions in the original protocol that are designed to run on a single cluster or metabolite. In order to apply WGCNA on the above datasets, the open source code base of WGCNA has been extended by additional utility functions. These extensions allows to circumvent the limitations that are hard to produce for non-computational experts (i.e. biologists) and hence cause them losing a lot of time and eventually interest in applying such advanced methods. As a result, an iterative method of WGCNA was developed which allows users with little or no coding skills to run the code in a swift and user-friendly manner. This improved strategy greatly expands the general applicability of WCGNA and provides processes that runs in a loop for deriving relating modules to external clinical traits and identifying important links between these traits and genes.
The final step in this project is to provide a Jupyter Notebook with SoS kernel suitable for executing WGCNA, MOFA and mixOmics in an user-friendly interface [Fig.1]. Jupyter Notebook is developed to easily run scripts by non-experts and to allow more reproducible analysis. The provided notebook includes a function to merge significant genes among the three methods to identify common genes.
Tags: bioinformatics |
Address
Rijvisschestraat 120
9052 Gent
Belgium |
Contacts
Traineeship supervisor
Alexander Botzki
0032 9 244 66 34 Alexander.Botzki@vib.be |
Oren Tzfadia
oren.tzfadia@vib.be |