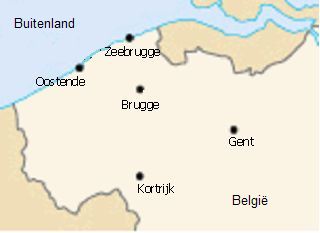
Pages
VIB-Ugent Center for Inflammation Research Charlotte Scott Lab
Abstract Traineeship 2020-2021: ANALYSIS OF CITE-SEQ DATA USING JOINT PROBABILISTIC MODELING
The hamster represents a useful model for biological research especially in light of the current Covid-19 pandemic as unlike mice, hamsters are susceptible to infection with the SARS-CoV-2 virus. Despite this, to date the cellular make up of different organs has not been studied in detail. This stems from a lack of useful antibodies with which the different cell types can be distinguished. With this in mind, the main biological goal of this research is to define different cell types and cell states in the spleen and lung tissue of healthy hamsters. Moreover, we are interested in assessing if any existing anti-mouse antibodies are cross-reactive with the cells from the hamster spleen and lung and thus could be used to characterize the immune response in this species following SARS-CoV-2 infection. To examine this, cells from lung and spleen tissue were isolated by enzymatic digestion of the tissues, stained with a pool of ~200 barcoded anti-mouse antibodies and sequenced in a method referred to as cellular indexing of transcripts and epitopes by sequencing (CITE- seq). This droplet-based sequencing method provides information on both mRNA and surface protein expression in each single cell, allowing the distinction in the different cell types and cell states and the identification of useful antibodies to further study these cells. While this is the ultimate goal of this study, my project was focused on assessing the packages used and their capabilities to analyze CITE-seq data. To this end, the R based Seurat package and the Python based TotalVI package were used to study the data.
During the first step of the pipeline, the mRNA data of 4 biological replicates was analyzed, using the Seurat R package. This step is designed to identify and remove all cells which do not meet the quality requirements. These requirements involve a deviating amount of genes, RNA or mitochondrial genes present in the cell. These aspects are indicators for doublets, poor quality cells and dying cells. After the removal, a log-normalization and a scaling step is executed. However, both these steps are not powerful enough to remove technical differences between samples (i.e. batch effect correction). For this the Harmony data integration was applied. Further, a PCA and UMAP dimensionality reduction analysis was performed. The results provided by the PCA and UMAP analysis are used to make an initial visualization of the clusters. This visualization alongside the list of differentially expressed genes per cluster allows the biologists to further identify unusual clusters, such as those resembling doublets, which are then removed from the dataset in further rounds of clean up.
While this process was sufficient for the spleen data, the results provided by the Seurat analysis suggested a significant amount of ambient mRNA in the samples. Specifically, many of the cells expressed the neutrophil gene S100a8. Ambient RNA is mRNA present in the supernatants of cell suspensions used for the sequencing analysis. This ambient RNA is then captured in droplets together with a cell’s mRNA despite the absence of the original cells, in this case, neutrophils, from which the ambient mRNA originates. To remove ambient RNA signals, the FastCAR package was used. After running the lung samples through FastCAR, the Seurat analysis and cleanup described above was performed to further clean the dataset.
After this thorough clean-up of the single cell data, the TotalVI analysis can be initiated. The algorithms provided by the TotalVI Python package were applied simultaneously on the mRNA and protein expression data. During the TotalVI analysis a model is made, which relies on neural networks and Bayesian methods. Neural networks belong to the artificial intelligence category, which mimic the way the human brain works. The method is used to classify non-linear data. The Bayesian method is a probabilistic modelling method, which classifies the data based on probability calculations. Based on the model, a low-reduction representation can be made from the data representing both mRNA and protein expression of the cells. The representation enhances the ability to correctly identify the cells. Additionally, the model is also utilized to denoise the data and to identify differential expression of RNA and proteins in different cells.
By following these steps, we can conclude that a thorough cleaning of the single cell data is crucial prior to performing a full analysis to ensure only real cells are studied. After removing ambient RNA, low quality and contaminating cells we were able to make an UMAP plot in TotalVI using the mRNA and surface protein expression simultaneously, this can be seen in figure 1 A and B, where the different clusters and the distribution of the different samples is shown. Different cell types could be identified together with genes unique to the cell types. In figure 1 C, D E and F, the expression of four different cell specific genes are shown. For example, in figure 1 E, an enlarged expression of the Cd3g gene can be seen. From those results, there can be suggested cluster 0, 14, 23 and 28 are T cells. In figure 1 F, the expression of C1qa is shown. From these results, there can be suggested that cluster 16 exists out of macrophages. For the surface proteins high background signals were detected, which makes it hard to identify surface proteins unique to the different cell types. This is probably caused by a low affinity between the mouse antibodies and the hamster surface proteins. Further investigation is needed how the parameters of the TotalVI model can be tweaked to remove the background signal as much as possible.
Tags: bioinformatics |
Address
Belgium |
Contacts
Traineeship supervisor
Liesbeth Martens
liesbet.martens@ugent.be |
Traineeship supervisor
Charlotte Scott
charlotte.scott@ugent.be |
|